Computer Science 210 Lab 4: Complexity and Slow Programs
Due: February 24, 1998
Objectives and Overview: This lab provides a chance to become
familiar with basic ideas of computational complexity, which is a way of
measuring the efficiency of programs in terms of the speed at which they
run. What makes a particular program the most efficient solution to a given
problem, and how do we know how efficient it is? You should use Chapter
5 in your text, along with your knowledge of the Vector class, as background
material for this lab.
Two specific problems, the "maximum subsequence sum" (MSS)
problem and the sorting problem, provide good vehicles for exploring the
subject of computational complexity.
Part 1 - Maximum Subsequence Sums
Four specific solutions to the MSS problem developed in Chapter 5 and
Chapter 7 can be easily exercised by using the MaxSum.cpp program.
This program in turn uses the Vector and Exception classes, whose .h and
.cpp files should be combined together in your Lab4.µ project (see
below):
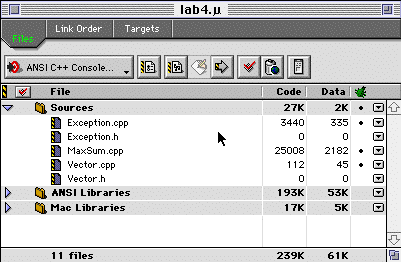
- Run this project by typing an array size of 10. What is the answer?
Check this answer by hand, using the 10 random numbers displayed on the
screen for this run.
- Run this project again for an array size of 100. Do you notice any
change in the behavior of the program? That is, does the program run quickly?
- Run this project four more times, for array sizes of 100, 200, 500,
and 1000. With your wristwatch, keep track of the approximate time it takes
to complete the entire run.
- Describe as precisely as you can what happened to the run time as the
array size increased in this way. Be careful to carefully contrast the
run times of the four different approaches to the MSS problem that the
program exercises.
- Briefly, how do your findings correspond to the "theoretical"
complexities of these four approaches to MSS, as discussed in class and
in your text?
Part 2 - Sorting
Here, we have an opportunity to explore the complexity of sorting, using
the sort function that you first exercised in Lab 1. This version of that
function uses the Vector class, and the main program keeps track of the
running time of the sort for various sizes of the input. Again, a random
number generator is used to build an unordered array of the appropriate
size, which is displayed both before and after the sort.
In the project you developed for Part 1, replace the MaxSum.cpp
program by the Sorter.cpp program, and remake the project.
- Now run this program for each of the following array sizes: 100, 500,
1000, 2000, 3000, 5000, and 10000. Record the elapsed time, in seconds,
reported for each run.
- How does the running time of the sort seem to grow as the array size,
x,g rows? That is, does the complexity of this sorting program seem to
be O(x)? O(x2)? O(x3)? O(xlog x)?
- How would you design an experiment that could measure the "best
case" performance of this sorting algorithm, in terms of forcing a
minimum number of calls to the function SwapRef?
- What about an experiment to test the "worst case" performance
of this sort?
- (Optional) If you have time, implement your "best case" and
"worst case" experiments and rerun the program using the array
sizes of question #1. How many calls to the function SwapRef are
executed for each of these cases, for the array size x=1000? What is the
actual difference in run time between "best case", "worst
case", and "average case" (represented by the original run
with random numbers)
Lab 4 Deliverables:
Hand in your answers to the questions in Parts 1 and 2 of this lab.
Also, hand in a hard copy of the output from a single run of the sorter.cpp
program, for an array size x = 100.